How data transformation drives margin growth realization
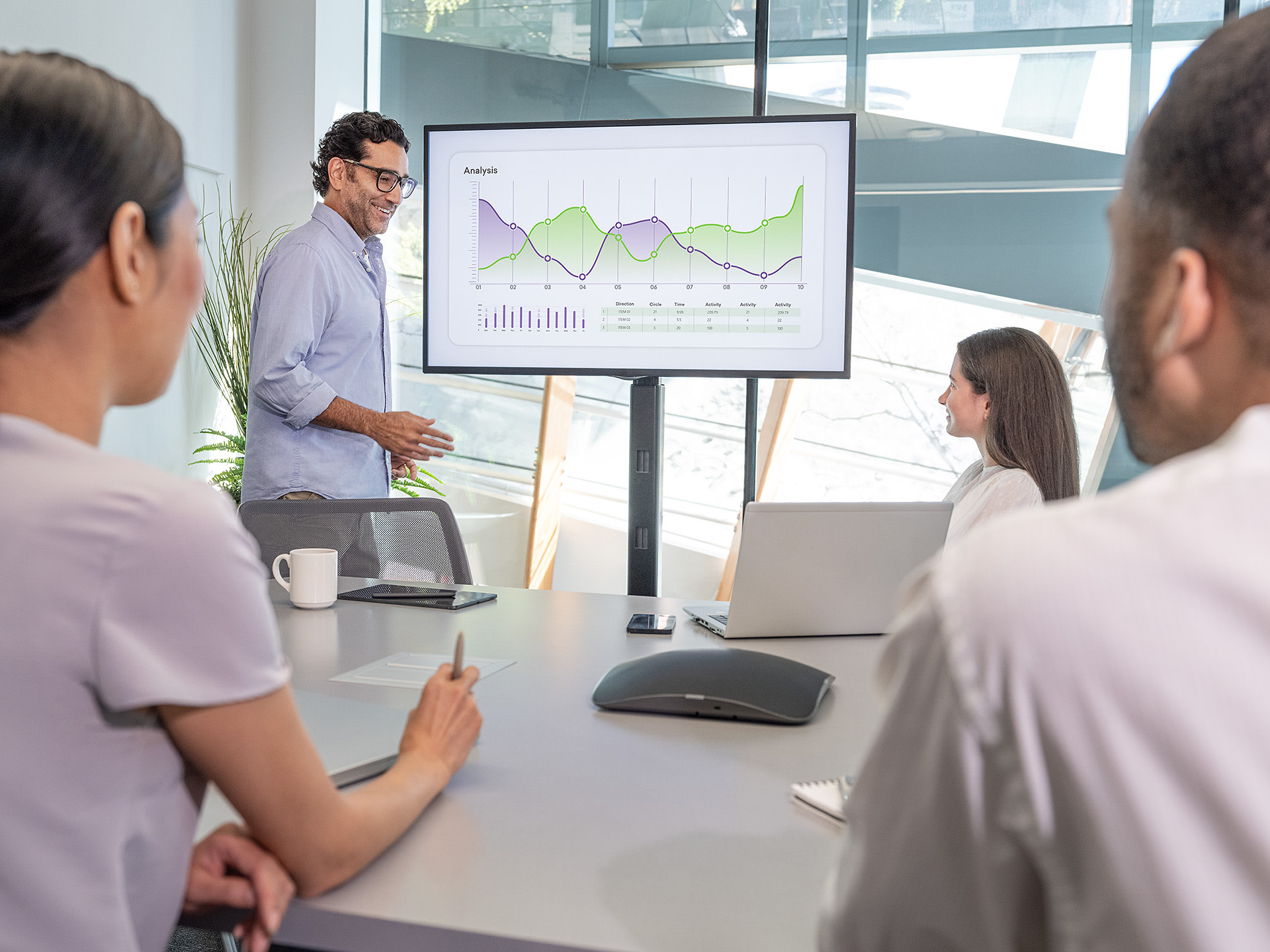
Today, data transformation is crucial for CPG manufacturers in retail and foodservice to boost margins and grow revenue. This is particularly important in the current economic climate where households are tightening their budgets due to rising costs. To navigate these challenges, manufacturers need to adapt their strategies, focusing on what we at TELUS call "margin growth realization" (MGR). This concept emphasizes the need to shift attention from volume and revenue to the bottom line and margin. MGR involves not only adapting Revenue Growth Management (RGM) strategies but also ensuring coordination between strategy and in-store compliance. This approach is essential for CPG companies to stay competitive and ensure long-term success in a drastically changed landscape where traditional focus on volume and revenue is no longer sufficient.
At a recent TELUS event, David Ganiear, a partner at PwC Strategy& shared, “Since exiting the pandemic, the CPG industry has faced unprecedented inflation. Manufacturers discovered that while prices increased, sales volume dropped. Now, they are focusing on building resilience for future challenges.” He added that in recent years, companies adopted a "field of dreams" strategy, building data warehouses and lakes with the hope that insights would follow. While this laid a foundation, it didn't drive the expected value. CFOs, CMOs, CIOs and CTOs experienced strained relationships due to significant tech investments without clear returns, highlighting the need for a more strategic approach to technology and data management.
In this blog, we will explore how effective data transformation and artificial intelligence can help drive margin growth realization, turning data investments into tangible business value.
CPG industry trends and challenges
The CPG industry is up against several challenges, like rising costs, shifting consumer behavior and the need for efficient supply chain management. Data transformation helps tackle these issues by harmonizing data to offer a unified view of data sets, enabling businesses to make better informed decisions more quickly.
Consumer behavior in retail
Household budgets are getting tighter, and consumers have less spending power. Meanwhile, the cost of goods keeps rising. This is changing how shoppers behave, with more value orientation and hunting for the best deals. They're finding ways to save by:
Looking for promotions and deals more often1
Postponing purchases until items go on sale1
Focusing only on essential purchases2
Skipping non-essential buys2
Switching from premium brands to private labels3
Upsizing to get better value packs3
Because of these factors, brand and retailer loyalty has dropped. Consumers are now frequently switching brands and shopping at different retailers to find the best prices.
Consumer behavior in foodservice
Customers are seeking value in foodservice, especially when dining out. They're:
Noticing price increases on everyday menu items3
Taking advantage of promotions to dine at off-peak times3
Feeling that portion sizes have gotten smaller3
Using restaurant loyalty apps for savings and rewards3
Abandoning delivery apps once they see the fees3
Looking for restaurants without credit card fees or surcharges3
With these consumer trends happening globally, it's more important than ever to start with data transformation on your journey to achieving margin growth realization.
Understanding data transformation
Simply put, data transformation is the process of harmonizing raw data from multiple sources and turning it into a format that's easier to understand and analyze. This process involves cleaning, structuring and integrating data to ensure it's high quality and ready for use. In the CPG industry, data transformation is key to understanding consumer behavior, optimizing supply chains and driving profitable growth.
Types of data transformation
There are several types of data transformation that data scientists can use to convert raw data into meaningful insights:
Data cleaning: Removes errors, duplicates and inconsistencies from the data set to ensure accuracy
Data integration: Combines data from different sources to provide a unified view
Data harmonization: Ensures that the integrated data is consistent and comparable. This involves standardizing data formats, units of measure and terminologies across different data sources to ensure that the data can be accurately compared and analyzed
Data aggregation: Summarizes data to provide a higher-level view, such as total sales by region or retailer
Data normalization: Adjusts data to a common scale without changing the differences between the values
Data enrichment: Enhances data by adding additional information from external sources
Benefits of data transformation: speed to insights and efficiency
Turning raw data into high-quality, actionable insights allows manufacturers to improve decision making, enhance operational efficiency, increase reporting accuracy and unlock advanced analytics. With full visibility into your data, you can understand how to alter a specific lever, like pricing or promotions, to align with consumer behavior.
Improve decision making
One of the main benefits of data transformation is the ability to make more informed decisions. With data sources harmonized, businesses can analyze trends, spot opportunities and use data to aid in decision making on the fly. Understanding consumer behavior helps you create targeted marketing strategies for each retailer or foodservice account. You can adjust prices and promotions based on what shoppers prefer.
Enhance operational efficiency
Data transformation boosts operational efficiency by streamlining business processes. Centralizing data reduces the time and effort needed to collect, harmonize and analyze information. This allows teams to focus on more strategic tasks, like developing new product launch plans. Additionally, integrated data helps optimize supply chains by identifying inefficiencies and areas for cost savings.
Increase reporting accuracy
Centralizing data helps enable teams within an organization to work with the same information, increasing reporting accuracy and reducing the risk of errors and discrepancies. For example, having a single source of truth for sales and inventory data can help prevent out of stocks and overstocks, leading to better inventory management.
Unlock advanced analytics
Data transformation unlocks advanced analytics and insights by providing a comprehensive view of data sets. This allows businesses to perform more sophisticated analyses, such as predictive modeling and trend forecasting. For instance, integrating sales data with market trends can help companies forecast demand and adjust their production schedules accordingly.
Implementing data transformation into business processes
Implementing data transformation in business processes involves several steps, from identifying data analysis sources to harmonizing and visualizing data. The result of data transformation is alignment between your technology and your business objectives. This sets the stage for achieving the ultimate goal of margin growth realization.
1. Identify and assess data sources
The first step in data transformation is to identify and assess all available data sources. This involves inventorying data sets and evaluating their current use and value to the business. By understanding what data is available and how it is used, manufacturers can find areas for improvement.
There are several types of data to consider:
Internal data: Generated by the manufacturer and includes proprietary information such as profit margins
Syndicated or vendor-aggregated data: Provided by third parties or brokers and includes retailer sales data for both your brand and competitor brands
Panel data: Shared by third parties and offers insights into consumer behavior, such as household penetration
Retail direct data: Distributed by the retailer and includes point-of-sale scan data
Distributor data: Provided by the distributor and contains shipment information from the distributor to the retailer
2. Implement data ingestion tools
Once you've identified your data sources, the next step is to implement data ingestion tools to collect and centralize the data. These tools handle various data formats and ensure data quality. It's important to plan ahead for the data intake process to accommodate new data sources and changes in data needs.
3. Regularly check for data ingestion errors
Regularly checking for data ingestion errors is important to ensure data accuracy and completeness. Consider spot checking to validate data as it comes in and addressing any issues immediately. Maintaining data quality makes your analyses and insights reliable, building trust within your team.
4. Integrate and harmonize data
To integrate and harmonize data, standardize definitions and terms to establish a consistent view across data mapping sources. This step makes data meaningful and useful within your organization. Clearly define harmonization standards, such as aligning with an enterprise resource planning tool or a shopper decision tree. Additionally, define the products, periods, markets and metrics that matter to your business.
5. Visualize data
Visualizing data allows you to understand and interpret your data quickly and efficiently. This involves creating high-quality reports and dashboards that provide actionable insights. To begin, align with stakeholders on a small number of reports, refine them and ingrain them in the business processes. Integrating data visualization with decision-making tools, such as TELUS Trade Promotion Management, can further enhance its value.
6. Iterative improvement
Unfortunately, this isn’t a set-it-and-forget-it task. Data transformation requires continuous refinement and improvement. Treat data centralization as an iterative process, regularly reviewing and updating your data transformation strategies to meet evolving business needs. Stay up to date with new data sources, technologies and best practices.
7. Maximize margins
You’ve completed your data transformation and built in steps for iterative improvement–so, what’s next? To help drive success, focus on margin growth realization. This involves integrating sales planning and execution across various tools.
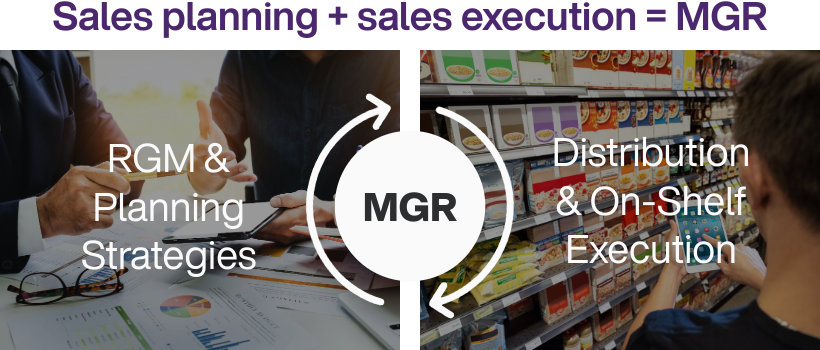
Margin growth realization helps to align revenue growth management strategies with execution and distribution solutions, driving perfect store execution to maximize margins. To begin a margin growth realization initiative, consider adding these tools to your tech stack:
TELUS Revenue Growth Management AnalyticsTELUS Trade Promotion ExcellenceTELUS Sales Enablement TELUS Retail ExecutionThe future of data transformation in the CPG industry
With all the tech advancements in data analytics, like machine learning and artificial intelligence (AI), it's an exciting time for the CPG industry. To keep up with changing consumer behavior and market conditions, leveraging data transformation and margin growth realization is becoming more important for manufacturers that want a competitive edge.
Leverage AI
AI is revolutionizing data transformation. By leveraging AI, businesses can automate business intelligence tasks such as data collection, cleaning and analysis, reducing the time and effort required to transform data. AI can also provide more sophisticated insights, such as identifying patterns and trends that may not be immediately apparent to the human eye.
Hesham Fahmy, Chief Information Officer at TELUS, emphasizes a crowd-sourced approach to AI integration: "At TELUS, we believe GenAI can be a game changer for our productivity, innovation and business competitiveness so we’re ramping up adoption across our global team and embedding AI into the very DNA of our organization, our culture and how we work. We have lowered the barriers to entry and have equipped our team with the latest, internal GenAI tools with built-in security and privacy controls so everyone can experiment with AI and learn new ways to be productive and innovative, no matter what their role." By integrating AI into products, like the TELUS TPM solution, TELUS equips individuals with tools to make more informed decisions at scale.
With boundless opportunities in the future, Fahmy adds: "We wouldn't be able to fully unlock the transformative possibilities of AI if we didn't have our foundational building blocks in place. Data is the lifeblood and fuel of any organization and having a strong data strategy is critical to developing and implementing AI successfully. Getting that right unlocks so much potential."
Capturing value through data transformation and AI
As the CPG industry continues to evolve, the importance of data transformation cannot be overstated. Ganiear's insights highlight the transition from merely investing in technology to strategically leveraging it for measurable value. “This shift in the industry has really gone from making investments, buying technology and generating the datasets to focusing on use cases that drive value. Demonstrating the upside strengthens the connection between the CIO and CFO.”
By embracing data transformation and focusing on strategic AI use cases, you can not only navigate current challenges but also position your company for sustained growth and success in an increasingly competitive market. This approach also helps weather future storms, such as another pandemic, by building resilience and adaptability into operations.
Margin growth realization may be achieved by coordinating sales planning and retail execution.
Book a demo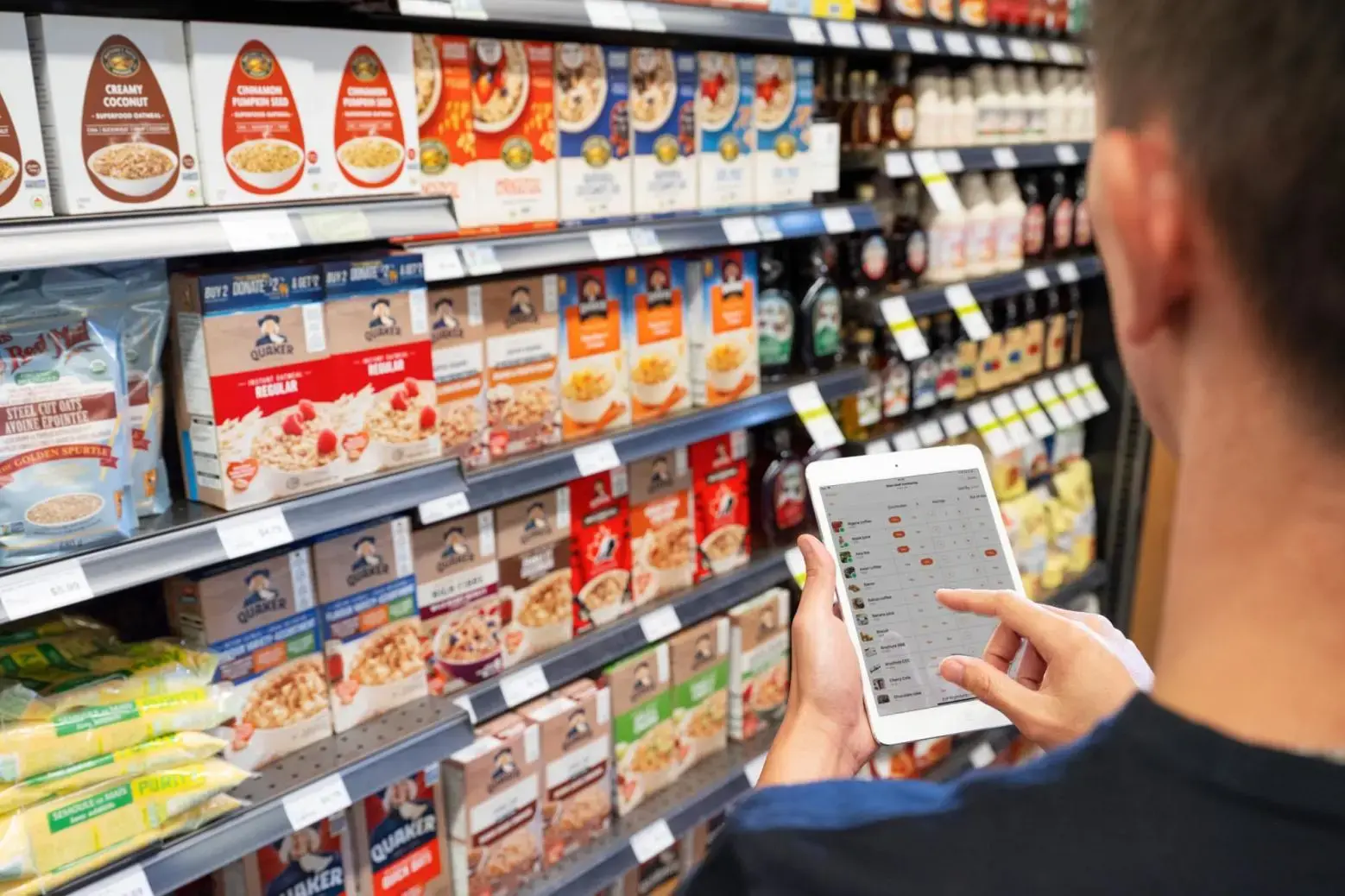
1 TELUS (2023). “11th Annual Food & Beverage Study.” 2 Opia (2023). “The promotion effect on shifting consumer behaviour.” 3 Oracle (2024). “9 Grocery Retail Trends in 2024.” 4 FoodBytes (2024). “2024 Food Trends.”